INST362 - SAVVY UX TEAM 12
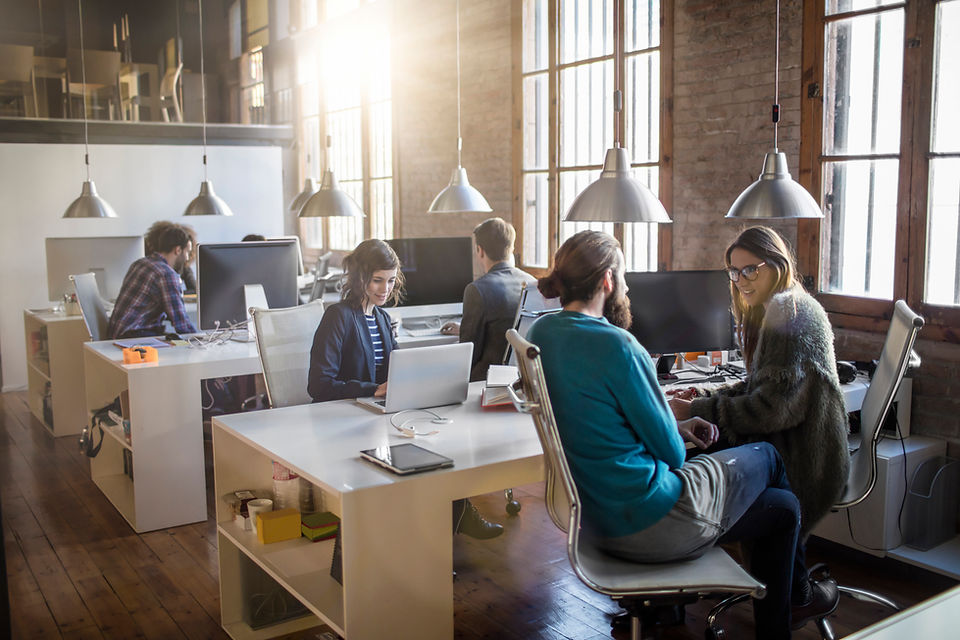
REPORT: ARTIFACTS AND OUTCOMES OF PROTOTYPE TESTING
PROCESS
We, as a group, individually presented those we interviewed in the past with the task of responding to a series of scenarios and providing feedback for our designs. Using powerpoint, we provided 16 scenarios with images as aid and asked how our participant would respond to the given situation. Would they use our idea of a pedestrian bridge, continue to cross the road as normal, or use any other method of crossing the street? Each scenario had conditions based on the weather, traffic density, and whether or not the participant is starting from inside or outside of the building they are crossing from. Based on the participants response, we gave a score of +1 point that would eventually be tallied at the end. The score of each decision, we can determine which method of crossing the street was the most popular and therefore most likely to be used.
Number of Participants: 8 people tested our prototypes
Each team member was tasked with conducting the test process with at least one participant they have previously interviewed. The results of each testing session were then combined into one document where the scores are translated into quantitative data from using Tableau, Weka, Open Refine, decision trees, and information gain calculations.
ANALYSIS
Weka:
From loading our combined scoring .csv file into Weka, we can see that the attribute of Path to Take shows 88 counts of ‘Yes’, which means that users crossed the bridge to get to the other side of the road, and 40 counts of ‘No’, which means that users just crossed the road to get to the other side.
We used Naive Bayes, a machine learning method, to run this dataset and calculate the probability of a class, which in this case the path to take (bridge), is predicted to occur, assuming that the attributes are independent of each other. The % of correctly classified instances is 87.5%. This is a good model. 87.5% of instances were correctly put in the right class, which is decently good. In the confusion matrix, this is okay because there are more correctly identified ‘a’ in class ‘a’ and ‘b’ in class ‘b’ than a wrong class identified in another class. The confusion matrix is quite balanced and predicts accurately about 90% of the time for no and 70% of the time for yes, which is good. True Positive Rate is fair, and False Positive Rate is low as class instances are mostly correctly classified. The area under the curve ROC Area, generally higher the better, and 0.904 is good. Also, the Kappa statistic is showing .6967 of the expected accuracy and the actual accuracy in the validation sets, which is above average. Finally, the Relative Absolute Error (RAE) is only 55.13% which isn’t the best as it is not close to 0, but at least much better than random guessing (which would be 100%) so it’s again fair.
OpenRefine:
We used OpenRefine for data wrangling, cleanup, and transformation on my dataset and grouped the values based on the path to take and the total case of scenarios. This allowed for an easier and cleaner way of viewing the data and how much of the values is filtered and displayed in each feature through grouping.
Tableau:
Using Tableau allowed us to group the data into an organized table of the four attributes and display a visualization showing the path to take with red for bridge and blue for crossing the road. From this bar chart, we can see an overwhelming response for taking the bridge to cross the road when the participant started inside and a split in choosing the bridge or road depending on the traffic and weather when started outside.
Decision Tree & Information Gain:
In conclusion from doing manual calculation of the information gain on the attributes traffic, location, and weather, we learned that since the number of bits for gain in location is greater than the gain in traffic and weather, the better gain is in Location (0.233 bits). In other words, location is more likely to influence users in their choice of taking a path.
FINDINGS
What We Learned?
- Most of our participants liked the idea of our plan where having a bridge being built as a way for crossing the road (ex. between two buildings like between Hornbake and ESJ building).
- According to the participants, the 16 scenarios mentioned during testing was helpful in knowing how and when the bridge would be used, depending on the change of weather, location, and traffic.
- Of the three factors in influencing the path to take in crossing the road, the factor location was surprisingly the most influential in determining how the participants crossed the road to the other side.
- Two major reasons why participants decided to cross the bridge was due to convenience, if they had started inside the building, and for shelter from the weather, to cross the road.
- When the scenario had rainy weather, all but one of the 32 responses chose the path of the bridge regardless of location and traffic condition
- More participants chose to cross the road when the weather was sunny or cloudy when they started outside than compared to when the weather was rainy or snowy when they started outside.
The participants provided more suggestions and preferences that could help to improve the design of the bridge upon looking at the images and drawings of examples.
- The preferences and suggestions include:
- Stairs over escalators as escalators require frequent maintenance and are large.
- Stairs that are u-shaped (half-turn) compared to straight as the u-shaped save space for being more compact.
- An overhang or ceiling over the bridge and stairs is preferred over having none because it can provide shelter from the weather and shade.
- Having an access ramp as replacement for stairs is more friendly for all who use, especially the disabled. A downside would be that it would take longer and more effort to go up or down the ramp as the distance
travelled is longer compared to standard stairs length of distance from bridge to sidewalk.
What are the recommended next steps for this team project?
- More testing for a broader and increased number of responses. As we only had 8 participants, who are around the same age, to test our prototype, it would be interesting to see what responses we would get from younger, older, and disabled participants.
- Pick a Finalized bridge design based on the suggestions from our participants and start to build a prototype.
How would we change or elaborate on your product?
- Theoretical recommendations include creating a more interactive prototype for a more interactive testing. As we are in a pandemic, we were unable to create a hands-on physical prototype for our participants to interact with and better understand our project. This would mean doing in-person testing, so we are able to show our models to the participants.
- Another recommendation could be to create an interactive 3D model online that can allow users to simulate being in a virtual environment and have them pick a path to choose to cross to the other side of the road while also being able to control the different attributes of different scenarios. This way, all participants can ‘live’ through the experience in each scenario and make more ‘accurate’ choices